Data FinOps Demystified: Unlocking secrets to your Data & AI ROI
As data cloud vendors enjoy a windfall, organisations are increasingly grappling with the challenges of scaling their data and analytics operations in a cost-effective manner.
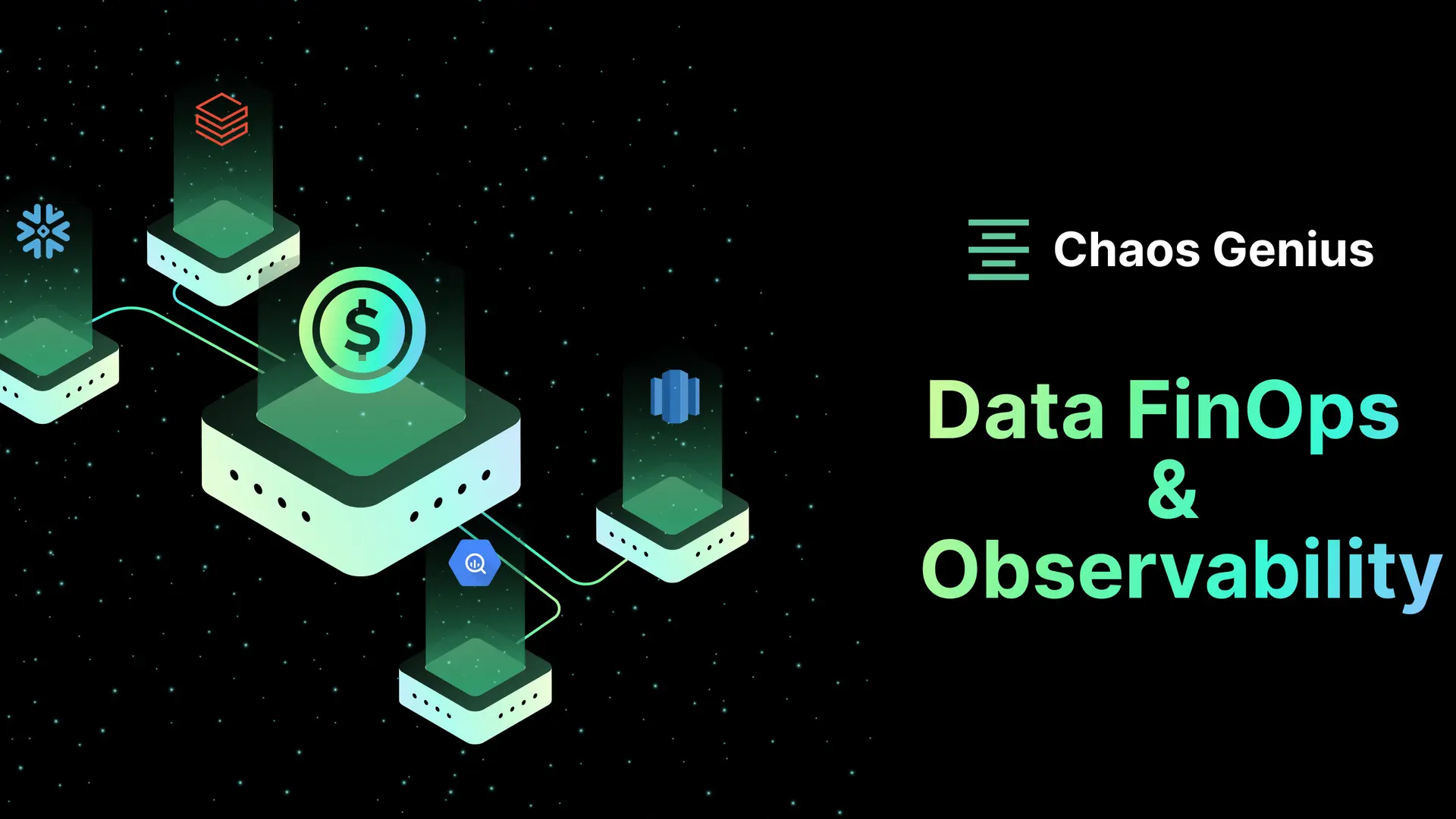
Humans and businesses alike have become increasingly data-driven over the last decade. You only need to look at how much data we generate on a daily basis (around 2.5 quintillion bytes) to recognise that.
While no single business is handling quite that volume of data, the general rise in data and need for insights has led to an increased requirement for big data solutions like cloud data warehouses/lakes . These warehouses and data lakes act as a data management system, enabling data teams to run various Business Intelligence (BI) and analytical activities.
However, on-premise systems are no longer enough. Businesses and their data teams continue to seek greater scalability alongside real-time data analytics and reporting functionalities. This has led to exploding demand for data clouds like Snowflake and Databricks in the last decade.
The great migration
For context, Snowflake is a cloud-based data storage and analytics platform that’s often wrapped up as a DaaS (Data-as-a-Service) solution. The product has great features like unlimited scalability of workloads via decoupled storage and compute resources, multi-cloud support to prevent vendor locking, high-performance queries, and security, among others.
Within just 12 years of founding, Snowflake (SNOW) boasts of being a $72 billion publicly traded company. It shares its key performance metrics every quarter, which mentions they’ve been able to migrate 647 of the Forbes Global 2,000 customers on to their platform while having ~$2.8 billion in run-rate revenue. That’s HUGE.
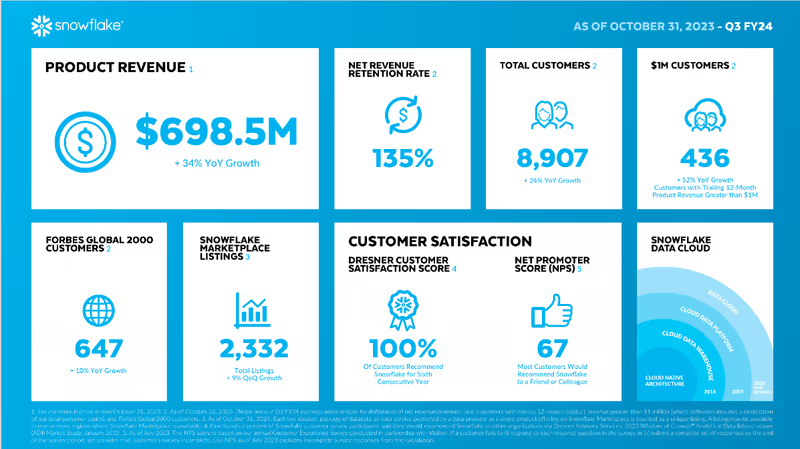
Similarly, another competing platform Databricks, which is a private company, is backed by some of the most prominent investors worldwide -Andreesen Horowitz, NVIDIA, Morgan Stanley, and Fidelity to name a few. Databricks was last valued at $43 billion. Databricks boasts of $1.5 billion in revenue run-rate, 10,000+ customers globally and 50% of the Fortune 500 on their platform.
The data costs question
As data cloud vendors enjoy a windfall, organisations are increasingly grappling with the challenges of scaling their data and analytics operations in a cost-effective manner. Between Databricks and Snowflake, there are over 750 organizations in the world spending more than one million dollars per year on these platforms.
Are these costs warranted? Yes and no.
Yes, because harnessing the power of data and AI is becoming imperative for organisations to innovate and stay competitive.
No, because the lack of tooling to monitor usage and optimise costs is leading to a high level of inefficiencies in these systems.
Three primary factors contribute to the escalating costs in data clouds: limited cost visibility and monitoring, poor warehouse utilisation, and inefficient queries.
Limited cost visibility and monitoring
Due to lack of inbuilt dashboards, it’s especially difficult for users to monitor their costs in a granular way and pinpoint the exact reason for cost increases. Without a clear view of the data cloud infrastructure and workloads, IT, data and finance teams are struggling to optimise performance and effectively manage costs.
Ineffective warehouse/cluster management
When cloud data warehouses are first set up, data teams may lack the know-how, time, or confidence to spawn instances with the most optimal settings. As a result, they end up relying on default settings suggested by these platforms, which are more often than not, very costly.
Inefficient workloads
In an organisation, there can be thousands of users running hundreds of millions of workloads on these systems. There’s no way to effectively analyse this usage and pinpoint the costliest workloads which are just poorly written code. Query tuning and workload optimisation is often done manually by data admin teams, which takes significant time away from their core job.
Navigating the storm with Data FinOps
Enter Data FinOps.
Creating efficiencies where inefficiencies once propagated is just one way that businesses and their data teams can get control of costs. Let’s look at what steps data teams should take to control expenses:
Understand current usage and cost
By using proper tooling, it’s possible to get granular insights into your data cloud usage across instances, users, teams and workloads. Bringing in this visibility is the first step towards optimising costs.
Implement cost-saving measures
Once the key cost drivers are identified, data teams can then go about optimising those. This can be done via different measures like Instance Right-Sizing to identify wasteful compute spend, workload optimisation to ensure efficient jobs, and, lastly, identifying unused data assets to manage storage costs.
The optimisation recommendations can be fetched using a new array of Data FinOps tools that harness the power of AI to monitor data systems and generate automated recommendations.
Enabling observability
To keep optimising ongoing data operations, it’s recommended to set up alerts to notify teams of unexpected spikes in usage or cost. This is an often overlooked aspect of Data FinOps, which leads to large unexpected cloud bills.
Implementing these efficiency measures can help businesses save up to 30%-50% on data cloud costs, which is certainly no small feat.
As a startup, ChaosGenius is leveraging this opportunity to develop a first-of-its-kind Data FinOps platform that uses AI to help companies manage their costs and performance across multiple data clouds, including Snowflake and Databricks. Chaos Genius is a self-serve platform that takes 10 minutes to set up and has been deployed in production in various Fortune 500 organisations, delivering up to 30% in savings and 20X return on investment for data teams.
Cohort 12 of NetApp Excellerator
Chaos Genius recently graduated from the latest cohort of the NetApp Excellerator Program. The DataOps Observability platform received mentorship and networking opportunities with potential clients and collaborators. The expertise and solutions offered by NetApp in hybrid cloud data services align perfectly with their needs.
Chaos Genius completed a successful proof-of-concept with NetApp, aiding the company in reducing its Snowflake data warehousing costs by 28% and delivering an impressive 20x return on investment within a mere 3 months.
(Disclaimer: The views and opinions expressed in this article are those of the author and do not necessarily reflect the views of YourStory.)
Preeti Shrimal is the Founder and CEO of Chaos Genius, a Data FinOps & Observability platform, backed by Y-Combinator and Elevation Capital.